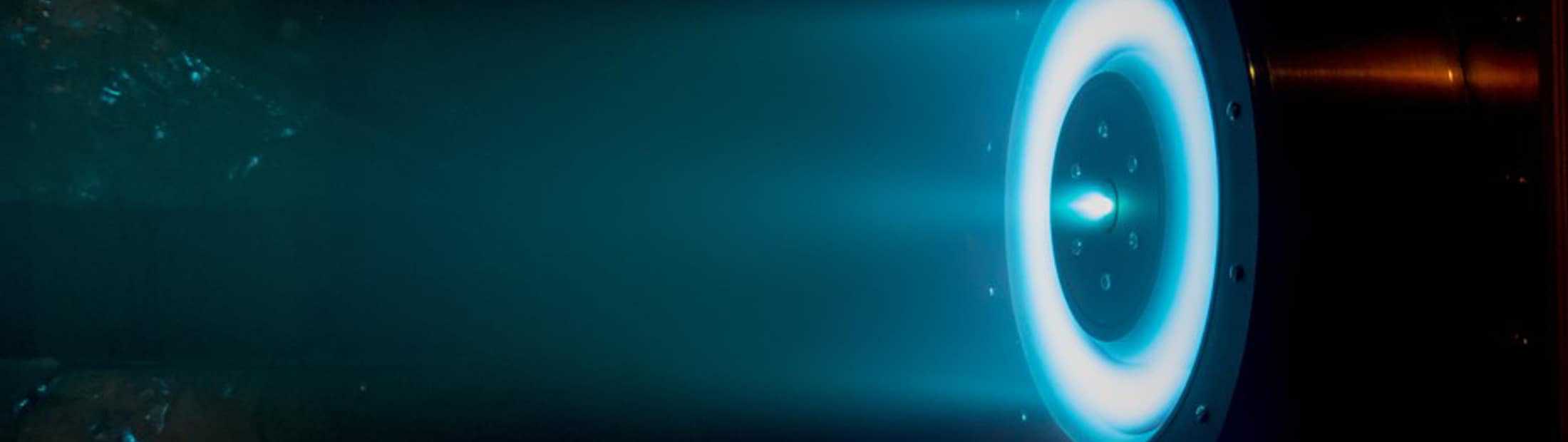
Jorns’ predictive Hall thruster model research receives Best Paper award
The data-driven approach to developing better predictive models will result in faster and higher-fidelity engineering models for advanced rocket propulsion systems.
The data-driven approach to developing better predictive models will result in faster and higher-fidelity engineering models for advanced rocket propulsion systems.
University of Michigan Aerospace Engineering Assistant Professor Benjamin Jorns won the 2020 Best Paper Award in Electric Propulsion from the AIAA Propulsion and Energy Forum conference for his paper “A predictive Hall thruster model enabled by data-driven closure.”
An expert in the experimental and theoretical study of Hall thrusters for in-space propulsion, Jorns received the same Best Paper recognition in 2016 and was co-author of the paper that won in 2019.
A Hall thruster is an electric rocket engine that traps electrons in a magnetic field and uses them to ionize the onboard propellant. The heavier species from this ionized propellant are then accelerated by strong electric fields.
A Hall effect thruster firing at the U-M Plasmadynamics and Electric Propulsion Laboratory. Jorns is using data-driven methods to develop predictive models for these devices.
More fuel efficient than conventional rocket engines, Hall thrusters are low-thrust devices that operate for thousands of hours and can accelerate their propellants to speeds higher than 20,000 mph. They have been widely used for commercial applications, most recently SpaceX’s StarLink Internet satellites. These devices also have been baselined for a number of deep-space missions.
According to Jorns, although Hall thrusters are a mature technology, some challenges remain in developing predictive models, which are required to more efficiently flight qualify and further develop the technology.
“There’s no computer program where you can dial in a brand new Hall thruster geometry and be confident that the predictions of the model will be indicative of what the actual performance will be,” said Jorns, who is co-director of the U-M Plasmadynamics and Electric Propulsion Lab.
Jorns’ approach to predictive modeling of Hall thrusters is based on machine learning and data-driven analysis. His award-winning paper is one of the first demonstrations of these methods in fully predictive thruster code.
Current U-M doctoral student Thomas Marks and recent graduate Dr. Ethan Dale were co-authors of the paper. Dale now works for the Aerospace Corporation. Their research was funded by the Air Force Office of Scientific Research (AFOSR) through its Space Propulsion and Power portfolio.