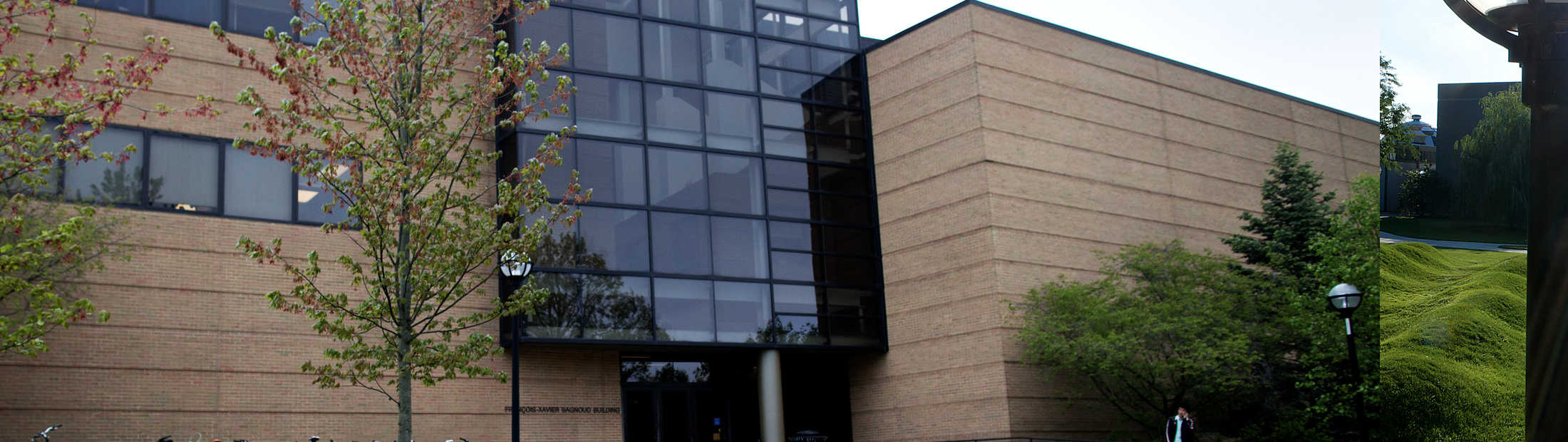
Two Aero PhD candidates win Predoctoral Fellowship
Gurmeet Singh and Kaiwen Liu acknowledged for their contributions
Gurmeet Singh and Kaiwen Liu acknowledged for their contributions
Two Aerospace PhD students were awarded coveted Rackham Predoctoral Fellowships this year. The funding, provided by the Rackham Graduate School, offers exceptional PhD students from across the university to finish their dissertations with funding to support their research and writing. Nominated by departments, the competitive funding program is awarded to students who’s dissertation abstracts, awards, publications and presentations showcase their exceptional contributions at U-M.
Aero’s recipients this year are Gurmeet Singh with a dissertation entitled “Multiscale Modeling of Vitrimers and Additively Manufactured Materials” and Kaiwen Liu writing about “Control and Learning Methods for Safe Autonomous Driving.”
Singh’s faculty advisor is Prof. Veera Sundaraghavan, where they research new composite materials in aircraft design. On receiving this funding, Singh comments, “I am thankful to the Rakham committee for considering me worthy of this prestigious award. I am also grateful to my PhD advisor, Prof. Veera Sundaraghavan, and the recommenders for supporting my application. The funding will help me work on my dissertation during the final stage of my PhD journey. This award will play a significant role in supporting my current research, and will bring me recognition and new possibilities towards my future endeavours.”
Singh’s dissertation summary:
Epoxies, used as binders in aircraft composites, are inherently non-recyclable. There has been tremendous interest in developing alternative epoxies that can be recycled. Vitrimers are one such promising system that combines recyclability with self-healing behavior, allowing sustainable use of composite materials. Despite the clear advantages, the mechanical response of vitrimers is not well characterized and this has limited its widespread use in aerospace applications. The thesis aims to address this gap by developing mechanical response models for vitrimers while solving a major challenge in vitrimer modeling which is accounting for dynamic cross-linking during thermal cycling. A topological reaction model was developed in which reaction templates are used along with a reaction rate model to initiate and equilibrate chemical reactions in molecular dynamics simulations. Self-healing and its effect on mechanical properties are modeled for the first time in literature, providing insights into the design of processing methods to improve mechanical properties.
Liu works under faculty advisor Professor Anouck Girard and Professor Ilya Kolmanovsky, focusing on modeling, control and learning methods for safe autonomous driving. Liu echoes the importance of this funding, stating, “Rackham Predoctoral Fellowship provides me with a great opportunity to stay focused on dissertation and improve the overall quality of my research and writings. The knowledge, insight, and skills gained from this focused research will also benefit my long-term career as a researcher.”
Liu’s dissertation summary:
This dissertation develops game-theoretic based decision frameworks to handle interactions between human-driven vehicle and autonomous vehicle (AV) and learning frameworks to operate AV in unknown circumstances, with emphasis on ensuring safety during the interaction and learning process. Firstly, predictive models for human interactions are developed based on game theory and a decision-making framework is proposed based on hybrid estimation and optimal control with explicit safety characterization. The effectiveness of the proposed approach is validated based on a comprehensive set of simulation-based case studies. Secondly, two learning schemes are developed for AV to autonomously explore its limits. The learning schemes rely on control theory and set theory, ensure the safety of AV when operating in unknown circumstances, and are verified based on several autonomous driving applications. The main contributions of this dissertation are developments of autonomous driving control and learning technologies that explicitly accounted for and ensured safety during their operations.